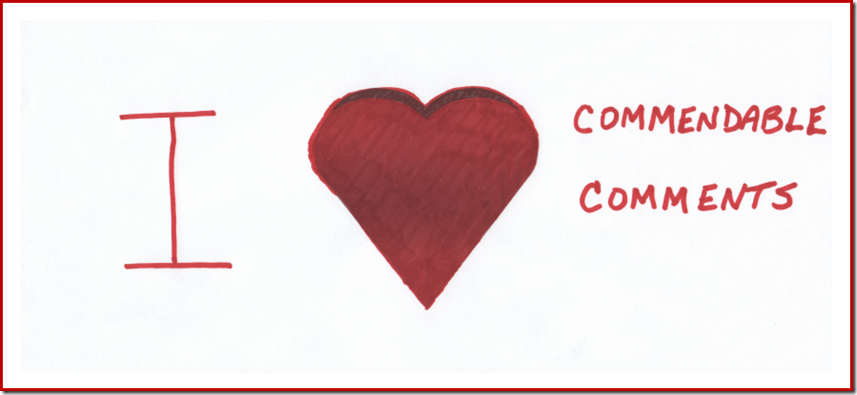
Today is February 14 — Valentine’s Day — the annual celebration of enduring romance, where true love is publicly judged according to your willingness to purchase chocolate, roses, and extremely expensive jewelry, and privately judged in ways that nobody (and please, trust me when I say nobody) wants to see you post on Twitter, Facebook, Flickr, YouTube, or your blog.
This is the ninth entry in my ongoing series for expressing my true love to my readers for their truly commendable comments on my blog posts. Receiving comments is the most rewarding aspect of my blogging experience. Although I love all of my readers, I love my commenting readers most of all.
Commendable Comments
On Data Quality Industry: Problem Solvers or Enablers?, Henrik Liliendahl Sørensen commented:
“I sometimes compare our profession with that of dentists. Dentists are also believed to advocate for good habits around your teeth, but are making money when these good habits aren’t followed.
So when 4 out 5 dentists recommend a certain toothpaste, it is probably no good :-)
Seriously though, I take the amount of money spent on data quality tools as a sign that organizations believe there are issues best solved with technology. Of course these tools aren’t magic.
Data quality tools only solve a certain part of your data and information related challenges. On the other hand, the few problems they do solve may be solved very well and cannot be solved by any other line of products or in any practical way by humans in any quantity or quality.”
On Data Quality Industry: Problem Solvers or Enablers?, Jarrett Goldfedder commented:
“I think that the expectations of clients from their data quality vendors have grown tremendously over the past few years. This is, of course, in line with most everything in the Web 2.0 cloud world that has become point-and-click, on-demand response.
In the olden days of 2002, I remember clients asking for vendors to adjust data only to the point where dashboard statistics could be presented on a clean Java user interface. I have noticed that some clients today want the software to not just run customizable reports, but to extract any form of data from any type of database, to perform advanced ETL and calculations with minimal user effort, and to be easy to use. It’s almost like telling your dentist to fix your crooked teeth with no anesthesia, no braces, no pain, during a single office visit.
Of course, the reality today does not match the expectation, but data quality vendors and architects may need to step up their game to remain cutting edge.”
On Data Quality is not an Act, it is a Habit, Rob Paller commented:
“This immediately reminded me of the practice of Kaizen in the manufacturing industry. The idea being that continued small improvements yield large improvements in productivity when compounded.
For years now, many of the thought leaders have preached that projects from business intelligence to data quality to MDM to data governance, and so on, start small and that by starting small and focused, they will yield larger benefits when all of the small projects are compounded.
But the one thing that I have not seen it tied back to is the successes that were found in the leaders of the various industries that have adopted the Kaizen philosophy.
Data quality practitioners need to recognize that their success lies in the fundamentals of Kaizen: quality, effort, participation, willingness to change, and communication. The fundamentals put people and process before technology. In other words, technology may help eliminate the problem, but it is the people and process that allow that elimination to occur.”
On Data Quality is not an Act, it is a Habit, Dylan Jones commented:
“Subtle but immensely important because implementing a coordinated series of small, easily trained habits can add up to a comprehensive data quality program.
In my first data quality role we identified about ten core habits that everyone on the team should adopt and the results were astounding. No need for big programs, expensive technology, change management and endless communication, just simple, achievable habits that importantly were focused on the workers.
To make habits work they need the WIIFM (What’s In It For Me) factor.”
On Darth Data, Rob Drysdale commented:
“Interesting concept about using data for the wrong purpose. I think that data, if it is the ‘true’ data can be used for any business decision as long as it is interpreted the right way.
One problem is that data may have a margin of error associated with it and this must be understood in order to properly use it to make decisions. Another issue is that the underlying definitions may be different.
For example, an organization may use the term ‘customer’ when it means different things. The marketing department may have a list of ‘customers’ that includes leads and prospects, but the operational department may only call them ‘customers’ when they are generating revenue.
Each department’s data and interpretation of it is correct for their own purpose, but you cannot mix the data or use it in the ‘other’ department to make decisions.
If all the data is correct, the definitions and the rules around capturing it are fully understood, then you should be able to use it to make any business decision.
But when it gets misinterpreted and twisted to suit some business decision that it may not be suited for, then you are crossing over to the Dark Side.”
On Data Governance and the Social Enterprise, Jacqueline Roberts commented:
“My continuous struggle is the chaos of data electronically submitted by many, many sources, different levels of quality and many different formats while maintaining the history of classification, correction, language translation, where used, and a multitude of other ‘data transactions’ to translate this data into usable information for multi-business use and reporting. This is my definition of Master Data Management.
I chuckled at the description of the ‘rigid business processes’ and I added ‘software products’ to the concept, since the software industry must understand the fluidity of the change of data to address the challenges of Master Data Management, Data Governance, and Data Cleansing.”
On Data Governance and the Social Enterprise, Frank Harland commented:
“I read: ‘Collaboration is the key to business success. This essential collaboration has to be based on people, and not on rigid business processes . . .’
And I think: Collaboration is the key to any success. This must have been true since the time man hunted the Mammoth. When collaborating, it went a lot better to catch the bugger.
And I agree that the collaboration has to be based on people, and not on rigid business processes. That is as opposed to based on rigid people, and not on flexible business processes. All the truths are in the adjectives.
I don’t mean to bash, Jim, I think there is a lot of truth here and you point to the exact relationship between collaboration as a requirement and Data Governance as a prerequisite. It’s just me getting a little tired of Gartner saying things of the sort that ‘in order to achieve success, people should work together. . .’
I have a word in mind that starts with ‘du’ and ends with ‘h’ :-)”
On Quality and Governance are Beyond the Data, Milan Kučera commented:
“Quality is a result of people’s work, their responsibility, improvement initiatives, etc. I think it is more about the company culture and its possible regulation by government. It is the most complicated to set-up a ‘new’ (information quality) culture, because of its influence on every single employee. It is about well balanced information value chain and quality processes at every ‘gemba’ where information is created.
Confidence in the information is necessary because we make many decisions based on it. Sometimes we do better or worse then before. We should store/use as much accurate information as possible.
All stewardship or governance frameworks should help companies with the change of its culture, define quality measures (the most important is accuracy), cost of poor quality system (allowing them to monitor impacts of poor quality information), and other necessary things. Only at this moment would we be able to trust corporate information and make decisions.
A small remark on technology only. Data quality technology is a good tool for helping you to analyze ‘technical’ quality of data – patterns, business rules, frequencies, NULL or Not NULL values, etc. Many technology companies narrow information quality into an area of massive cleansing (scrap/rework) activities. They can correct some errors but everything in general leads to a higher validity, but not information accuracy. If cleansing is implemented as a regular part of the ETL processes then the company institutionalizes massive correction, which is only a cost adding activity and I am sure it is not the right place to change data contents – we increase data inconsistency within information systems.
Every quality management system (for example TQM, TIQM, Six Sigma, Kaizen) focuses on improvement at the place where errors occur – gemba. All those systems require: leaders, measures, trained people, and simply – adequate culture.
Technology can be a good assistant (helper), but a bad master.”
On Can Data Quality avoid the Dustbin of History?, Vish Agashe commented:
“In a sense, I would say that the current definitions and approaches of/towards data quality might very well not be able to avoid the Dustbin of History.
In the world of phones and PDAs, quality of information about environments, current fashions/trends, locations and current moods of the customer might be more important than a single view of customer or de-duped customers. The pace at which consumer’s habits are changing, it might be the quality of information about the environment in which the transaction is likely to happen that will be more important than the quality of the post transaction data itself . . . Just a thought.”
On Does your organization have a Calumet Culture?, Garnie Bolling commented:
“So true, so true, so true.
I see this a lot. Great projects or initiatives start off, collaboration is expected across organizations, and there is initial interest, big meetings / events to jump start the Calumet. Now what, when the events no longer happen, funding to fly everyone to the same city to bond, share, explore together dries up.
Here is what we have seen work. After the initial kick off, have small events, focus groups, and let the Calumet grow organically. Sometimes after a big powwow, folks assume others are taking care of the communication / collaboration, but with a small venue, it slowly grows.
Success breeds success and folks want to be part of that, so when the focus group achieves, the growth happens. This cycle is then repeated, hopefully.
While it is important for folks to come together at the kick off to see the big picture, it is the small rolling waves of success that will pick up momentum, and people will want to join the effort to collaborate versus waiting for others to pick up the ball and run.
Thanks for posting, good topic. Now where is my small focus group? :-)”
You Are Awesome
Thank you very much for sharing your perspectives with our collablogaunity. This entry in the series highlighted the commendable comments received on OCDQ Blog posts published in October, November, and December of 2010.
Since there have been so many commendable comments, please don’t be offended if one of your comments wasn’t featured.
Please keep on commenting and stay tuned for future entries in the series.
By the way, even if you have never posted a comment on my blog, you are still awesome — feel free to tell everyone I said so.
Related Posts
Commendable Comments (Part 8)
Commendable Comments (Part 7)
Commendable Comments (Part 6)
Commendable Comments (Part 5)
Commendable Comments (Part 4)
Commendable Comments (Part 3)
Commendable Comments (Part 2)
Commendable Comments (Part 1)