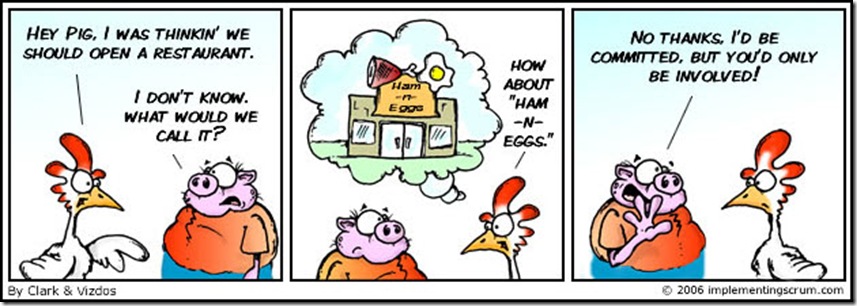
This was the inaugural cartoon on Implementing Scrum by Michael Vizdos and Tony Clark, which does a great job of illustrating the fable of The Chicken and the Pig used to describe the two types of roles involved in Scrum, which, quite rare for our industry, is not an acronym, but one common approach among many iterative, incremental frameworks for agile software development.
Scrum is also sometimes used as a generic synonym for any agile framework. Although I’m not an expert, I’ve worked on more than a few agile programs. And since I am fond of metaphors, I will use the Chicken and the Pig to describe two common ways that scrums of all kinds can easily get screwed up:
- All Chicken and No Pig
- All Pig and No Chicken
However, let’s first establish a more specific context for agile development using one provided by a recent blog post on the topic.
A Contrarian’s View of Agile BI
In her excellent blog post A Contrarian’s View of Agile BI, Jill Dyché took a somewhat unpopular view of a popular view, which is something that Jill excels at—not simply for the sake of doing it—because she’s always been well-known for telling it like it is.
In preparation for the upcoming TDWI World Conference in San Diego, Jill was pondering the utilization of agile methodologies in business intelligence (aka BI—ah, there’s one of those oh so common industry acronyms straight out of The Acronymicon).
The provocative TDWI conference theme is: “Creating an Agile BI Environment—Delivering Data at the Speed of Thought.”
Now, please don’t misunderstand. Jill is an advocate for doing agile BI the right way. And it’s certainly understandable why so many organizations love the idea of agile BI. Especially when you consider the slower time to value of most other approaches when compared with, following Jill’s rule of thumb, how agile BI would have “either new BI functionality or new data deployed (at least) every 60-90 days. This approach establishes BI as a program, greater than the sum of its parts.”
“But in my experience,” Jill explained, “if the organization embracing agile BI never had established BI development processes in the first place, agile BI can be a road to nowhere. In fact, the dirty little secret of agile BI is this: It’s companies that don’t have the discipline to enforce BI development rigor in the first place that hurl themselves toward agile BI.”
“Peek under the covers of an agile BI shop,” Jill continued, “and you’ll often find dozens or even hundreds of repeatable canned BI reports, but nary an advanced analytics capability. You’ll probably discover an IT organization that failed to cultivate solid relationships with business users and is now hiding behind an agile vocabulary to justify its own organizational ADD. It’s lack of accountability, failure to manage a deliberate pipeline, and shifting work priorities packaged up as so much scrum.”
I really love the term Organizational Attention Deficit Disorder, and in spite of myself, I can’t help but render it acronymically as OADD—which should be pronounced as “odd” because the “a” is silent, as in: “Our organization is really quite OADD, isn’t it?”
Scrum Screwed Up: All Chicken and No Pig
Returning to the metaphor of the Scrum roles, the pigs are the people with their bacon in the game performing the actual work, and the chickens are the people to whom the results are being delivered. Most commonly, the pigs are IT or the technical team, and the chickens are the users or the business team. But these scrum lines are drawn in the sand, and therefore easily crossed.
Many organizations love the idea of agile BI because they are thinking like chickens and not like pigs. And the agile life is always easier for the chicken because they are only involved, whereas the pig is committed.
OADD organizations often “hurl themselves toward agile BI” because they’re enamored with the theory, but unrealistic about what the practice truly requires. They’re all-in when it comes to the planning, but bacon-less when it comes to the execution.
This is one common way that OADD organizations can get Scrum Screwed Up—they are All Chicken and No Pig.
Scrum Screwed Up: All Pig and No Chicken
Closer to the point being made in Jill’s blog post, IT can pretend to be pigs making seemingly impressive progress, but although they’re bringing home the bacon, it lacks any real sizzle because it’s not delivering any real advanced analytics to business users.
Although they appear to be scrumming, IT is really just screwing around with technology, albeit in an agile manner. However, what good is “delivering data at the speed of thought” when that data is neither what the business is thinking, nor truly needs?
This is another common way that OADD organizations can get Scrum Screwed Up—they are All Pig and No Chicken.
Scrum is NOT a Silver Bullet
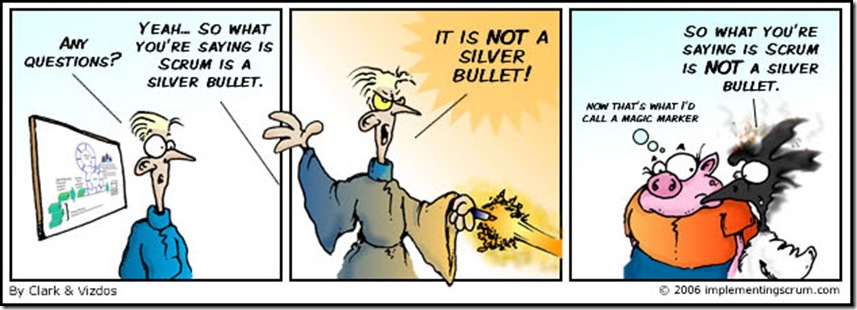
Scrum—and any other agile framework—is not a silver bullet. However, agile methodologies can work—and not just for BI.
But whether you want to call it Chicken-Pig Collaboration, or Business-IT Collaboration, or Shiny Happy People Holding Hands, a true enterprise-wide collaboration facilitated by a cross-disciplinary team is necessary for any success—agile or otherwise.
Agile frameworks, when implemented properly, help organizations realistically embrace complexity and avoid oversimplification, by leveraging recurring iterations of relatively short duration that always deliver data-driven solutions to business problems.
Agile frameworks are successful when people take on the challenge united by collaboration, guided by effective methodology, and supported by enabling technology. Agile frameworks allow the enterprise to follow what works, for as long as it works, and without being afraid to adjust as necessary when circumstances inevitably change.
For more information about Agile BI, follow Jill Dyché and TDWI World Conference in San Diego, August 15-20 via Twitter.