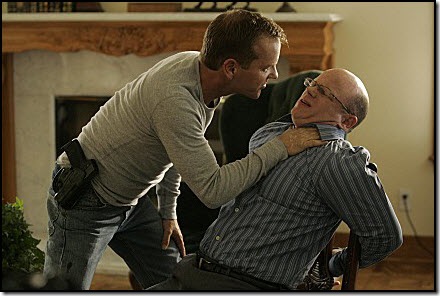
In my recent blog post Red Flag or Red Herring?, I explained that the primary focus of data governance is the strategic alignment of people throughout the organization through the definition, and enforcement, of policies in relation to data access, data sharing, data quality, and effective data usage, all for the purposes of supporting critical business decisions and enabling optimal business performance.
Simply establishing these internal data governance policies is often no easy task to accomplish.
However, without enforcement, data governance policies are powerless to affect the real changes necessary.
(Pictured: Jack Bauer enforcing a data governance policy.)
Jack Bauer and Data Governance
Jill Wanless commented that “sometimes organizations have the best of intentions. They establish strategic alignment and governing policies (no small feat!) only to fail at the enforcement and compliance. I believe some of this behavior is due to the fact that they may not know how to enforce effectively, without risking the very alignment they have established. I would really like to see a follow up post on what effective enforcement looks like.”
As I began drafting this requested blog post, the first image that came to my mind for what effective enforcement looks like was Jack Bauer, the protagonist of the popular (but somewhat controversial) television series 24.
Well-known for his willingness to do whatever it takes, you can almost imagine Jack explaining to executive management:
“The difference between success and failure for your data governance program is the ability to enforce your policies. But the business processes, technology, data, and people that I deal with, don’t care about your policies. Every day I will regret looking into the eyes of men and women, knowing that at any moment, their jobs—or even their lives—may be deemed expendable, in order to protect the greater corporate good.
I will regret every decision and mistake I have to make, which results in the loss of an innocent employee. But you know what I will regret the most? I will regret that data governance even needs people like me.”
Although definitely dramatic and somewhat cathartic, I don’t think it would be the right message for this blog post. Sorry, Jack.
Enforcing Data Governance Policies
So if hiring Jack Bauer isn’t the answer, what is? I recommend the following five steps for enforcing data governance policies, which I have summarized into the following simple list and explain in slightly more detail in the corresponding sections below:
- Documentation – Use straightforward, natural language to document your policies in a way everyone can understand.
- Communication – Effective communication requires that you encourage open discussion and debate of all viewpoints.
- Metrics – Truly meaningful metrics can be effectively measured, and represent the business impact of data governance.
- Remediation – Correcting any combination of business process, technology, data, and people—and sometimes, all four.
- Refinement – You must dynamically evolve and adapt your data governance policies—as well as their associated metrics.
Documentation
The first step in enforcing data governance policies is effectively documenting the defined policies. As stated above, the definition process itself can be quite laborious. However, before you can expect anyone to comply with the new policies, you first have to make sure that they can understand exactly what they mean.
This requires documenting your polices using a straightforward and natural language. I am not just talking about avoiding the use of techno-mumbo-jumbo. Even business-speak can sound more like business-babbling—and not just to the technical folks. Perhaps most important, avoid using acronyms and other lexicons of terminology—unless you can unambiguously define them.
For additional information on aspects related to documentation, please refer to these blog posts:
Communication
The second step is the effective communication of the defined and documented data governance policies. Consider using a wiki in order to facilitate easy distribution, promote open discussion, and encourage feedback—as well as track all changes.
I always emphasize the importance of communication since it’s a crucial component of the collaboration that data governance truly requires in order to be successful.
Your data governance policies reflect a shared business understanding. The enforcement of these policies has as much to do with enterprise-wide collaboration as it does with supporting critical business decisions and enabling optimal business performance.
Never underestimate the potential negative impacts that the point of view paradox can have on communication. For example, the perspectives of the business and technical stakeholders can often appear to be diametrically opposed.
At the other end of the communication spectrum, you must also watch out for what Jill Dyché calls the tyranny of consensus, where the path of least resistance is taken, and justifiable objections either remain silent or are silenced by management.
The tyranny of consensus is indeed the antithesis of the wisdom of crowds. As James Surowiecki explains in his excellent book, the best collective decisions are the product of disagreement and contest, not consensus or compromise.
Data Governance lives on the two-way Street named Communication (which, of course, intersects with Collaboration Road).
For additional information on aspects related to communication, please refer to these blog posts:
Metrics
The third step in enforcing data governance policies is the creation of metrics with tangible business relevance. These metrics must be capable of being effectively measured, and must also meaningfully represent the business impact of data governance.
The common challenge is that the easiest ones to create and monitor are low-level technical metrics, such as those provided by data profiling. However, elevating these technical metrics to a level representing business relevance can often, and far too easily, merely establish their correlation with business performance. Of course, correlation does not imply causation.
This doesn’t mean that creating metrics to track compliance with your data governance policies is impossible, it simply means you must be as careful with the definition of the metrics as you were with the definition of the policies themselves.
In his blog post Metrics, The Trap We All Fall Into, Thomas Murphy of Gartner discussed a few aspects of this challenge.
Truly meaningful metrics always align your data governance policies with your business performance. Lacking this alignment, you could provide the comforting, but false, impression that all is well, or you could raise red flags that are really red herrings.
For additional information on aspects related to metrics, please refer to these blog posts:
Remediation
Effective metrics will let you know when something has gone wrong. Francis Bacon taught us that “knowledge is power.” However, Jackson Beck also taught us that “knowing is half the battle.” Therefore, the fourth step in enforcing data governance policies is taking the necessary corrective actions when non-compliance and other problems inevitably arise.
Remediation can involve any combination of business processes, technology, data, and people—and sometimes, all four.
The most common is data remediation, which includes both reactive and proactive approaches to data quality.
Proactive defect prevention is the superior approach. Although it is impossible to truly prevent every problem before it happens, the more control that can be enforced where data originates, the better the overall quality will be for enterprise information.
However, and most often driven by a business triage for critical data problems, reactive data cleansing will be necessary.
After the root causes of the data remediation are identified—and they should always be identified—then additional remediation may involve a combination of business processes, technology, or people—and sometimes, all three.
Effective metrics also help identify business-driven priorities that determine the necessary corrective actions to be implemented.
For additional information on aspects related to remediation, please refer to these blog posts:
Refinement
The fifth and final step is the ongoing refinement of your data governance policies, which, as explained above, you are enforcing for the purposes of supporting critical business decisions and enabling optimal business performance.
As such, your data governance policies—as well as their associated metrics—can never remain static, but instead, they must dynamically evolve and adapt, all in order to protect and serve the enterprise’s continuing mission to survive and thrive in today’s highly competitive and rapidly changing marketplace.
For additional information on aspects related to refinement, please refer to these blog posts:
Conclusion
Obviously, the high-level framework I described for enforcing your data governance policies has omitted some important details, such as when you should create your data governance board, and what the responsibilities of the data stewardship function are, as well as how data governance relates to specific enterprise information initiatives, such as master data management (MDM).
However, if you are looking to follow a step-by-step, paint-by-numbers, only color inside the lines, guaranteed fool-proof plan, then you are going to fail before you even begin—because there are simply NO universal frameworks for data governance.
This is only the beginning of a more detailed discussion, the specifics of which will vary based on your particular circumstances, especially the unique corporate culture of your organization.
Most important, you must be brutally honest about where your organization currently is in terms of data governance maturity, as this, more than anything else, dictates what your realistic capabilities are during every phase of a data governance program.
Please share your thoughts about enforcing data governance policies, as well as your overall perspectives on data governance.
Follow OCDQ
If you enjoyed this blog post, then please subscribe to OCDQ via my RSS feed, my E-mail updates, or Google Reader.
You can also follow OCDQ on Twitter, fan the Facebook page for OCDQ, and connect with me on LinkedIn.