In the past, I have explained various aspects of data quality using blog posts inspired by two primary sources of wisdom:
1. Literature
2. Science (including Science Fiction)
However, in this blog post I want to channel the seldom tapped wisdom of Lloyd Christmas and Harry Dunne, from the Academy Award Eligible and American Cinema Classic – Dumb and Dumber.
The Dumb and Dumber Guide to Data Quality

“What the one doesn't have, the other is missing.”
Data and Quality—do they really need each other?
The Business and IT—do they really need to work together?
Isn't data quality an IT issue? After all, the data is stored in databases and applications that they manage. Therefore, if there are problems with the data, then IT is responsible for cleaning up their own mess. Aren't they?
Isn't data quality a Business issue? After all, the data is created by business processes and users that they manage. Therefore, if there are problems with the data, then the Business is responsible for cleaning up their own mess. Aren't they?
Listening to the Business and IT argue like this reminds me of Lloyd and Harry playing the game of Tag:
Lloyd: “You're it.”
Harry: “You're it.”
Lloyd: “You're it, quitsies!”
Harry: “Anti-quitsies, you're it, quitsies, no anti-quitsies, no startsies!”
Lloyd: “You can't do that!”
Harry: “Can too!”
Lloyd: “Cannot, stamp it!”
Harry: “Can too, double stamp it, no erasies!”
Lloyd: “Cannot, triple stamp, no erasies, touch blue make it true.”
Harry: “No, you can't do that . . . You can't triple stamp a double stamp! Lloyd!”
Lloyd [with hands over his ears]: “LA-LA LA-LA LA-LA!”
Harry: “LLOYD! LLOYD! LLOYD!”
Yes, the Business usually owns the data and understands its meaning and use in the day-to-day operation of the enterprise. And yes, IT usually owns the hardware and software infrastructure of the enterprise's technical architecture.
However, neither the Business nor IT alone has all of the necessary knowledge and resources required to truly be successful. Data quality requires that the Business and IT forge an ongoing and iterative collaboration.
Tag—you're both it! And executive management says: No quitsies!
Not every theory looks good in a tuxedo
“Hey, look, The Monkees. They were a huge influence on The Beatles.”
Without question, there are many theories available about how to properly execute a data quality initiative.
You read about them in critically acclaimed books. You hear about them in expert presentations at major industry conferences. You even sometimes see them published in blog posts underneath pictures of two weird looking dudes wearing wacky tuxedos.
Most theories include models describing an organization's evolution through a series of stages intended to measure its capability and maturity, tendency toward being reactive or proactive, and inclination to be project-oriented or program-oriented.
I am certainly an advocate of searching for sound theory and working with proven methodology.
But the harsh reality is there is no “one theory to rule them all” or one-size-fits-all methodology—and anyone who tells you otherwise should be treated with the same disdain as those who truly believe The Monkees were a huge influence on The Beatles.
You need to find something that will adapt to your organization's unique culture. Most important, you need to find something that will meet your organization wherever it happens to currently be within the capability and maturity model.
Just because some expert says you should be wearing a black Armani tuxedo with a crimson cummerbund and monogrammed cufflinks, doesn't mean you should. Maybe the bright orange or powder blue tuxedo with the frilly shirt, top hat, and cane is more your style. Or maybe it is the only thing currently in your size—or the only thing you can currently afford.
Rock whatever tuxedo (theory) fits you best today. Just remember—it's a rental. As your organization and the individual change agents leading the way mature and evolve, your wardrobe (culture) will become ready to evolve right along with it.
Only you can decide what theory works best for your organization—as well as when you're ready to take it to the next level.
Not every practice can be considered best
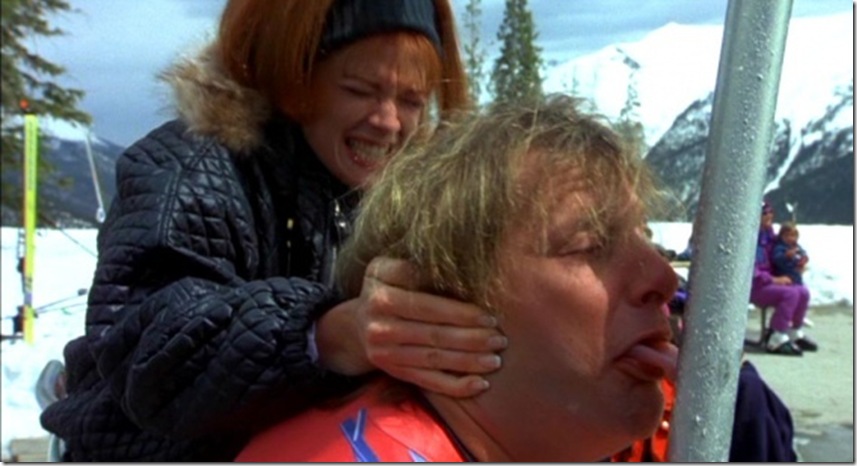
“Well, it's not gonna do us any good sitting here whining about it. We're in a hole. We're just going to have to dig ourselves out.”
So you have selected a theory and now you're ready to get to work. Every theory includes some recommended best practices.
However, if you are looking to follow a step-by-step, paint-by-numbers, only color inside the lines, fool-proof plan, then you are going to fail before you even begin.
Best practices simply provide a reference of recommended options of what proved successful for other data quality initiatives.
Best practices should be reviewed in order to determine what can be learned from them, as well as to select what you think will work in your environment and what simply won't. However, it often won't be easy to tell the difference.
The key word in “best practice” is practice—and not best, as in the perfectly stupid phrase: “practice makes perfect.”
Real practice doesn't make perfect. Real practice is messy. Real practice colors with the red crayon much more often than with the green crayon. Real practice doesn't color inside the lines—it draws on the walls.
In other words, you're going to make mistakes—lots and lots and lots—of mistakes.
And not because you are dumb—or dumber than others who successfully followed the same recommendations.
Not even best practices make perfect because nobody works at a company called Perfect, Incorporated. Through trial and error you will figure out what works best for you and your organization, and those practices will become your best practices.
Couldn't we get by just fine without data quality?
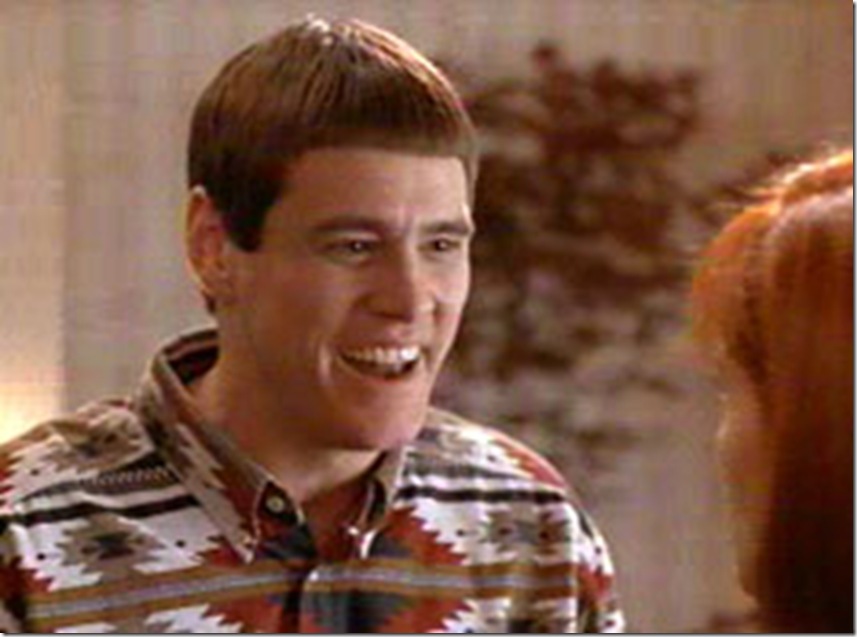
“So you're telling me there's a chance...”
You may be thinking that a data quality initiative sounds like a lot of work. You may be wondering if it is really worth the investment of all that time, effort, and money. You may be asking if you really need a data quality initiative.
Couldn't we get by just fine without data quality?
The following dialogue between Lloyd and Mary Swanson provides a better answer than anything else I can imagine:
Lloyd: “What do you think the chances are of us getting by just fine without data quality?”
Mary: “Well, Lloyd, that's difficult to say. I mean, we don't really...”
Lloyd: “Hit me with it! Just give it to me straight! What are the chances?”
Mary: “Not good.”
Lloyd: “You mean not good like one out of a hundred?”
Mary: “I'd say more like one out of a million.”
Lloyd: “So you're telling me there's a chance...”